Running Docker Command From Svelte
What we’re trying to do
I have a setup where different docker containers are used in conjunction to provide a single application. More specifically, a database, a sveltekit app, and (at the moment just one) container to run specific analyses. The idea is that the sveltekit app in container 1 triggers a script in container 2 to change data in the database in container 3.
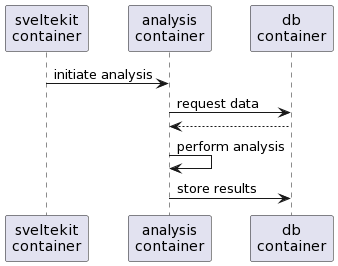
Directory structure
I created the following directory structure for the app:
docker-compose.yml
analysis/
Dockerfile
run_analysis.py
frontend/
Dockerfile
build/
public/
node_modules/
src/
...
The analysis
container only contains a Dockerfile
and the specific command to run the analysis; the frontend
container holds the svelte-kit code.
Setting up the analysis container
The key in this solution is the shell2http
script available from https://github.com/msoap/shell2http. This script enables you to attach a port on your machine to a specific script. For example, the command shell2http /ps "ps aux"
will return the output of ps aux
to your webbrowser if you go to http://localhost:8080/ps.
Let’s for example say that we have an ArangoDB collection in which we want to add a new key/value pair for the document with the id 1234
. The following simple python script does this.
|
|
Testing shell2http
The regular way of running the script (let’s name it run_analysis.py
) would be python ./run_analysis.py
. Therefore, we can make it available using shell2http
with the following command: shell2http /analysis "python run_analysis.py"
. This will return the following:
2022/07/03 11:45:03 register: /analysis (python2 run_analysis.py)
2022/07/03 11:45:03 register: / (index page)
2022/07/03 11:45:03 listen http://localhost:8080/
It can now be executed by going to http://localhost:8080/analysis.
Creating the Dockerfile
To get this to work from docker, we’ll start from an ubuntu base, install go, shell2http and python, and copy our analysis script. The CMD
needs to run the actual ssh2http command.
|
|
Setting up the svelte-kit container
Similarly, we can set up the svelte-kit application. It doesn’t matter here what that thing looks like, as long as we can go to the link show above somewhere (i.e. http://localhost:8080/analyse).
The code below is taken from the src/routes/__layout.svelte
file. It uses sveltestrap to incorporate bootstrap into the application, and shows a navigation bar with three links, including Analyse
. This one will go to the URL that we specified above and which triggers the shell2http script in the other container.
|
|
Creating the Dockerfile
The Dockerfile for this svelte-kit application is the following:
|
|
Docker compose
Let’s now combine everything into a single application, using docker compose. The docker-compose.yml
file sets everything up:
|
|
We can run everything with docker-compose up --build
.
This docker-compose.yml
file has 3 sections, one for each docker container.
For the analysis
and frontend
sections, the build
part defines in which directory we can find the Dockerfile for the analysis container. We also have to expose the port 8080 in the analysis
container, and forward the internal port 3000 to port 5050 for the frontend
(i.e. svelte-kit) container. So if we go to http://localhost:5050, it will actually go to port 3000 inside the container. Finally, we add the analysis
and frontend
container to the app-network
network. This will allow us to refer to these containers from the other ones by their name.
We don’t build the arangodb
container ourselves, but use the one from dockerhub. In volumes
we describe where on our local machine the data is stored so that it is preserved whenever we have to restart containers.
That’s it.